In the realm of 3D data analysis and model creation, the act of segmentation emerges as a cornerstone process, critical in the accurate interpretation and utilization of data. Segmentation, in its essence, involves partitioning a digital image or 3D scan into multiple segments (sets of pixels or voxels, respectively) to simplify its representation into something that is more meaningful and easier to analyze. This is particularly crucial when distinguishing between objects of interest and their surroundings, including the containers they might be held in or any adjacent objects they might touch. An illuminating example of the importance of precise segmentation can be observed through the analysis of a metal water container (flask) and its water content shown later in this article.
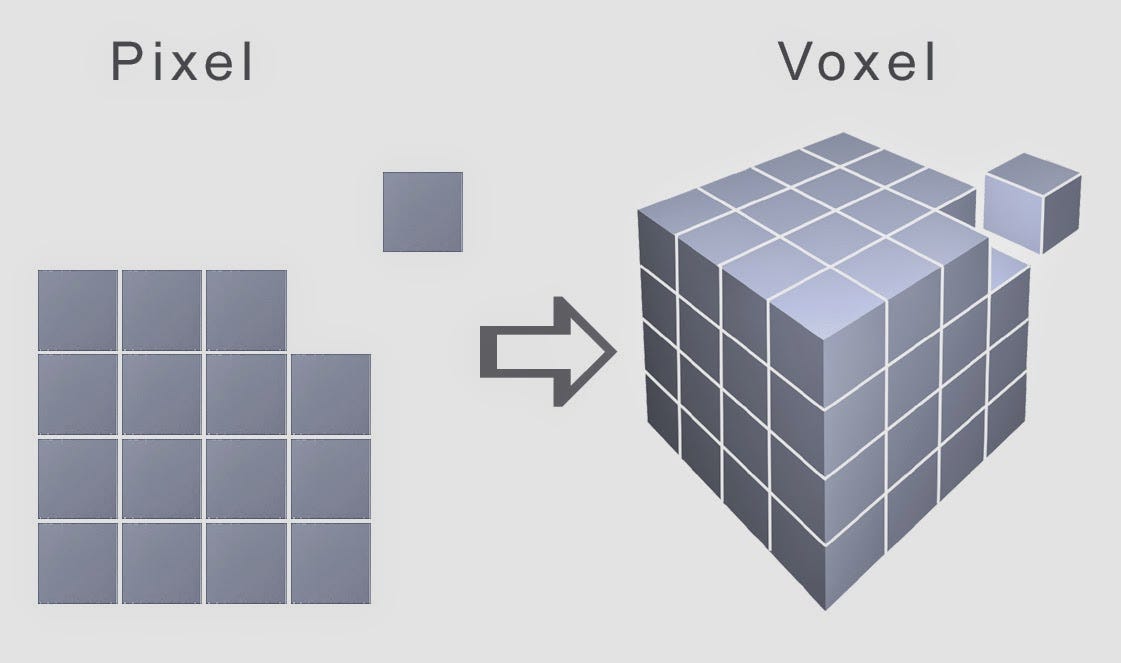
The image below shows a metallic hydro-flask with water with a couple of other objects nearby in a 3D CT scan. While you can make out the threats of the water bottle top and the outline of the container, you can observe the translucence nature of the object as it spins and realize that easily separating the container from its contents is no easy task. Let's use this scan as an example about how Stratovan's Segmentation for Pro-Surgical 3D can better prepare training data and help models and algorithms train to identify objects of interest.
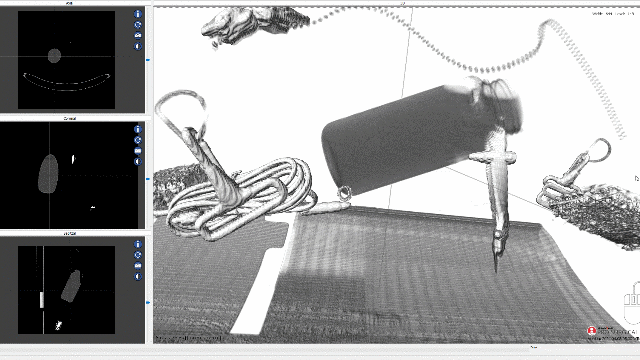
Imagine you're tasked with developing an algorithm capable of detecting and quantifying the amount of water in different containers from 3D scan data. In the real world, this exercise can be true of any material or liquid that exists within a container, but the example data we have is specific to liquid water in a metal drinking flask. The precision with which you can segment the water from the container itself becomes paramount. An accurate segmentation ensures that the algorithm can correctly identify and measure only the water, excluding the container. However, if the segmentation process allows for errors, such as bleed (where the segmentation spills over the boundary of the object) or overlaps onto surrounding objects, the result can significantly skew the algorithm's performance. Some objects of interest are bare, or alone, and don't have to worry about this issue as frequently. However, anytime your scans consist of complex and varied components, there will be a need to differentiate between objects you want to be identified, their surrounding neighbor objects, and any containers or materials they may be in contact with. The ramifications of this can have both massive and subtle impacts on the model and algorithm performance as it trains to not just search for the object you've segmented correctly, but any of the surrounding materials or containers marked in error.
In the image below, we start with the container itself. Now, as a hydro-flask, this object may not merit special attention or be worth segmenting on its own. However, if a company wants to put a product through trials and stress testing, and then scan for any changes in the structure, they could be interested in quality assurance testing to detect changes in the shape or wearing of the metal in places that might cause a leak. Additionally, if this flask were able to obscure and hide it's contents, security screening regulators and vendors might choose to segment this object to classify it as an obscuring object with the potential to house some threat or illegal material within. Whatever the case, it is important to have the tools at hand to be able to segment all types of objects in any scenario so you can prepare automated detection tools accordingly.
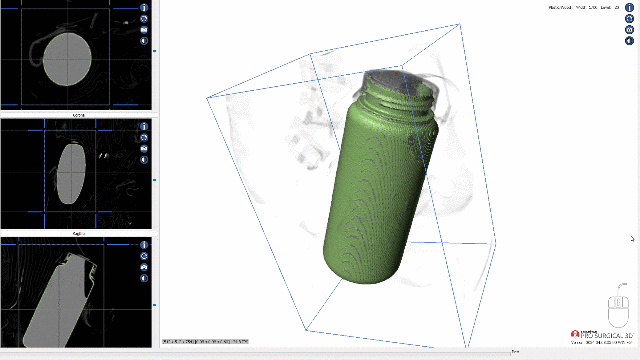
Segmentation errors introduce inaccuracies into the training datasets used to teach algorithms how to recognize and differentiate between objects. In our example, if the 3D scans of the water flask consistently include parts of the flask's metal in the segment designated as 'water,' the algorithm learns incorrectly. It might then generalize that reflective, metallic properties are characteristic of water, or it might misinterpret the shape and volume of water because its understanding includes part of the flask. Such inaccuracies lead to flawed model development, where the algorithm cannot reliably distinguish between the container and its content, or between the object of interest and nearby objects it touches. Without enough data to counteract this training error, an algorithm may only be able to identify water in a metal container, or it may create false detections on other metal containers without water, or fail to properly segment the water at all.
The consequences of these inaccuracies extend beyond just the immediate performance of the detection algorithm. They have tangible impacts on cost and time efficiency. Consider the application of this technology in industries where precise quantities of a substance are critical, such as pharmaceuticals, food and beverage, chemical manufacturing or threat detection. An algorithm that cannot accurately distinguish between substances because of poor segmentation can lead to incorrect ingredient amounts, affecting product quality, safety, and compliance with regulations. Correcting these errors post-production incurs significant costs and delays, highlighting the importance of accurate segmentation from the outset. On the other end, detecting dangerous compounds regardless of what they might be contained in can be the difference between a successful security screening operation and a horrific disaster.
Moreover, in fields such as medical imaging, the implications of inaccurate segmentation can extend to misdiagnoses and incorrect treatments, underlining the critical nature of precision in this process. Accurate segmentation ensures that algorithms are trained on data that truly represent the real-world distinctions between different materials and objects, leading to reliable, efficient, and safe applications across a wide range of industries. The human body is a complex place with different structures and materials all wrapped into one body. Training data segmentations need to be accurate within the millimeter to ensure the algorithm understands the differences between bones, blood vessels, skin, organs, and foreign entities without confusing one of those for the other and affecting positive detection and false detection rates.
The next image below showcases the water within the bottle successfully segmented. You can see that the water bottle is at an angle by the air within the container and how the liquid within comes to rest in such a way that the air pocket is not solely around the top, or the threaded area, of the bottle. This segmentation would allow a model to develop an understanding of how to detect water amongst a handful of other objects in luggage. Additionally, when combined with the container segmentation above, the model is better informed to understand that both the metal container and the water are two distinct and separate items of interest, and with enough data, be able to detect the presence of both in a 3D scan.
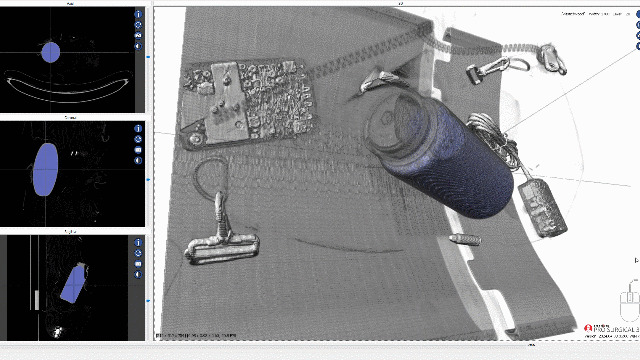
The process of refining segmentation techniques to eliminate errors and improve accuracy is ongoing. It involves sophisticated algorithms, including deep learning models, that can learn from vast amounts of data to distinguish between very subtle differences in texture, shape, and other characteristics. However, the quality of the outcome heavily relies on the quality of the input data. Therefore, the initial steps in data preparation, such as ensuring accurate segmentation of 3D data, are critical.
In summary, the example of segmenting the water content from a metal flask illustrates a broader principle in data analysis and model development. Accurate segmentation is not merely a technical requirement but a foundational step that can significantly influence the cost-effectiveness, time efficiency, and reliability of the algorithms developed from 3D data. It underscores the principle that in the realm of data science and machine learning, the quality of the inputs decisively shapes the quality of the outcomes. The phrase "garbage in, garbage out" directly refers to the quality and accuracy of training data, mainly the precision and consistency of segmented training data, and the impact it can have on algorithm performance outcomes. If you invest little time and effort, and segment quickly instead of with intentional quality, you can only expect as good of performance as the amount of investment you've placed in your data preparation efforts. As such, investing time and resources in precise segmentation is not just beneficial, but essential for developing algorithms that perform effectively and deliver real-world value.
To try Stratovan's Segmentation for Pro-Surgical 3D Software Suite for free, click here: https://www.stratovan.com/products/segmentation-pro-surgical-3d
If you're interested in having the data experts at Stratovan assist with a project, contact us here: https://www.stratovan.com/contact